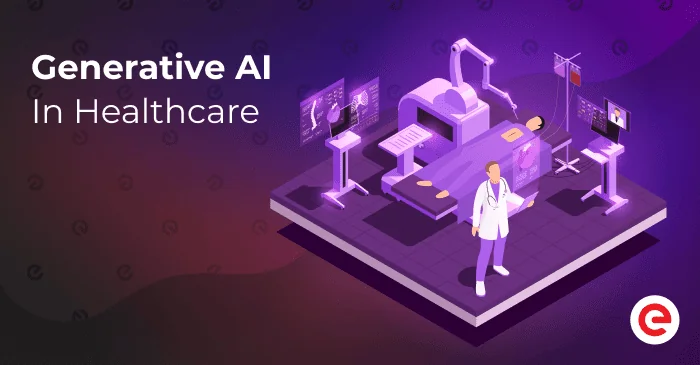
Updated: May 10, 2024
Published: October 18, 2023
Recent breakthroughs in the field of AI have expressed a great interest in adopting AI-powered solutions across various industries. The healthcare domain isn’t an exception, as it has always been among the first to leverage the latest approaches and technologies. In this article, we’ll discuss the topic of generative AI in healthcare and how it’s transforming this vital industry.
List of the Content
- What is generative AI?
- How is generative AI transforming healthcare?
- Generative AI examples in the medical industry
- Benefits of generative AI for healthcare
- How to adopt generative artificial intelligence in healthcare?
- Challenges of generative AI in healthcare
- Conclusion
WHAT IS GENERATIVE AI?
Over the recent years, the entire market has experienced AI’s transformative capabilities. With the growing need for continuous advancements, businesses find it efficient to leverage various tech solutions, including generative AI. In particular, it’s explained by the splash that powerful generative AI models have made lately. Even taking into account the buzz around ChatGPT, companies feel a necessity to keep the market pace as well as introduce something extra for their customers.
In the fastly evolving market, cutting-edge solutions are required across all industries and domains. Let’s start with the definition and explanation of generative AI and then move to its adoption in healthcare. It’s important to emphasize that generative AI has been around for years. But why is it drawing much more attention these days?
The decisive fact is that this technology has considerably surged forward. It was a long way from early, limited models to sophisticated, versatile systems. In the earlier versions of generative AI, developers had to submit data through APIs or other complex approaches. With advancements driven by research breakthroughs, increased computing power, larger datasets, and innovative model architectures, it introduced sophisticated generative AI models.
What is generative AI?
It refers to a type of artificial intelligence system and models designed to generate new data. The distinct characteristic is that generative AI isn’t designed for predictions or pattern recognition like with traditional AI systems. Indeed, it focuses on creating new data in the form of text, images, code, and more.
At this point, we can’t consider generative AI as something new in the tech world. However, the latest advancements are quite impressive. The market has been offered new AI capabilities and attracted the attention of businesses from different industries. ChatGPT (a large language model-based chatbot), DALL-E (text-to-image models), Llama 2 (an open-source large language model), Bard (a conversational generative artificial intelligence chatbot), and others have topped the list of popular generative AI applications.
Despite the solution specialization, the core task of generative AI remains to return new outputs in response to the provided prompts. It supports several overarching goals that vary depending on the specific application and context. Some of the primary purposes of generative AI include:
Content generation: Everything starts with the fundamental goal of creating new data, whether it’s text, images, audio, or other forms of content that are similar to what humans might produce.
Improved efficiency: In some cases, businesses find it efficient to automate tasks that would be time-consuming or labor-intensive for humans.
Personalization: Generative artificial intelligence can tailor content to individual preferences and needs.
Problem-solving: It is commonly used to solve complex problems, such as optimization tasks and generating solutions or suggestions.
Simulations and training: These AI models are also applied to simulate real-world scenarios for training purposes.
Research and scientific discovery: It can assist researchers by generating hypotheses, simulating experiments, and exploring scientific data to discover patterns and insights.
Human-AI collaboration: It goes without saying that this solution increasingly complements human capabilities. It can work alongside humans in tasks from fostering collaboration to decision-making.
As a result, we can find more and more domains to consider generative AI for their business-specific needs. Healthcare has also been among the first ones to leverage these cutting-edge solutions.
HOW IS GENERATIVE AI TRANSFORMING HEALTHCARE?
Continuous advancements in medical technology, pharmaceuticals, and treatment methods improve patient outcomes and drive progress in various fields of science and technology. What’s more, generative AI use cases in healthcare can be even more sophisticated compared to ChapGPT, Llama 2, or other trending AI apps.
According to Gartner, $1.7 billion has been invested in generative AI solutions by venture capital companies over the past three years. Much to the surprise of the market, AI-powered drug discovery and AI coding get the most funding. It means that proceeding to the discussion of generative AI in healthcare is really topical. While demanding special effort and careful consideration, it offers great potential for the industry.
The thing is that generative AI models receive the capability to process extensive medical data and generate fresh content, which has the potential to enhance the quality of healthcare, including its accessibility and affordability. The latest advancements can make it more applicable and suitable for various use cases. Besides the validated solutions, it’s worth drawing attention to its prospective adoption. Some conceptual ideas could quickly evolve and find adoption in real-world applications. It’s hard to predict how fast it would be implemented, but many teams specialize in advancing generative AI for healthcare.
The medical industry is undergoing lots of changes and transformations these days. Moreover, it refers to every healthcare segment due to numerous potential use cases.
- Healthcare providers use generative AI to enhance patient care, optimize their operations, accelerate medical research, and ultimately improve the overall healthcare experience for both patients and healthcare professionals.
- Pharmaceutical companies gain the potential to transform their domain and lead to the discovery of more effective treatments for various diseases. AI adoption accelerates the drug development process, improves the quality and safety of medications, reduces costs, and stays competitive in the rapidly evolving healthcare landscape.
- Public health agencies utilize this technology in a variety of ways to enhance their ability to monitor, respond to, and mitigate public health issues and challenges, from infectious disease outbreaks to chronic health conditions and environmental health risks. It enables data-driven decision-making and proactive responses to protect and improve public health.
- MedTech companies are projected to grow generative AI adoption for better healthcare services, personalized patient experiences, and cutting-edge innovations. It plays a pivotal role in advancing wearable health technology, rehabilitation and assistive devices, surgical assistance, etc.
- Healthcare payers, including insurance companies and government healthcare agencies, use generative AI to improve operational efficiency, manage costs, enhance member experiences, and make data-driven decisions. These technologies enable payers to adapt to evolving tendencies and provide better services to their members while managing financial sustainability.
The impact of generative AI in healthcare is multifaceted as it embraces all segments and helps to transform this industry for the advantage of every involved party.
GENERATIVE AI EXAMPLES IN HEALTHCARE
With the expanded range of generative AI use cases in healthcare, it’s interesting to discover new solutions in the market. These cutting-edge products and services improve the chances of significant advancements in the quality, affordability, and accessibility of medical care. Let’s consider some of the great generative AI examples in healthcare.
Med-PaLM 2
The progress in the development of LLMs supports numerous capabilities in language comprehension and generation. Thus, it has unveiled fresh opportunities to apply generative AI in healthcare to address real-world challenges. One of the great examples is Med-PaLM, an expert-level medical LLM powered by Google. This solution focuses on generating long-form, high-quality answers to health questions. Also, it was the first AI system to surpass the pass mark on USMLE-style questions. According to the latest updates, it has already achieved an accuracy of 86.5% with the Med-PALM 2 version. Generative AI in healthcare has definitely moved beyond pattern recognition and enables valuable content creation. Furthermore, it is interesting to note that they also work on extending beyond language and incorporating data on EHRs, sensors, images, wearables, etc.
AWS HealthScribe
The next example is the newly introduced HIPAA-eligible service introduced by Amazon Web Services. HealthScribe embraces the distinctive functionality of the automatic generation of clinical notes. Powered by generative AI and speech recognition, it helps healthcare providers save time by handling clinical documentation. It’s applicable for building clinical apps with AI-generated notes based on patient-clinical conversations. This solution seems to be an advancing tool to enhance productivity and boost scribe workflow. It’s also efficient for holding patient consultations, especially for powering quality consultations within healthcare apps.
Oracle Clinical Digital Assistant
Another generative AI healthcare service was launched in September 2023. That is Oracle Clinical Digital Assistant, powered by their existing EHR solutions. Oracle is a strong market player known for enhancing medical care experiences and acquires the largest EHR market share. They introduced this service based on generative artificial intelligence and voice commands. That primarily aims to reduce the manual tasks for healthcare providers. It makes it possible to engage the AI-powered assistant during appointments to automate notes, reduce errors, propose context-aware actions, order medication, schedule follow-up appointments, etc. As a result, it guarantees the full engagement of healthcare professionals and draws their focus to patient-centricity services.
Azure Health Bot
We can’t forget one of the top AI market players, Microsoft. It is definitely worth attention with its Azure OpenAI Service offering powerful AI models to create intelligent apps. Besides, we can find many other solutions specially delivered for the medical industry. For instance, Azure Health Bot enables developers within healthcare institutions to create and implement AI-driven, compliant, and scalable healthcare interactions. It integrates a built-in medical knowledge base with natural language capabilities to comprehend medical terminology. The solution also ensures compliance with industry regulations and maintains privacy standards in line with HIPAA. Health Bot allows providers to integrate with external systems and ease customization capabilities. It’s commonly used to provide self-serve functionality and enable access to required medical data.
Exploring generative AI use cases in healthcare can provide valuable insights, inspire innovation, and contribute to improving medical services and patient outcomes.
Would you like to discuss your idea for the AI-powered solution?
You’re welcome to contact our development team. Let us know your needs to find the best suitable software to cover your needs and deliver cutting-edge products and services.
BENEFITS OF GENERATIVE AI IN HEALTHCARE
Remaining a critical field, the medical industry can greatly benefit from advancements in AI technology. Generative artificial intelligence exploits the potential to revolutionize how healthcare professionals diagnose, treat, and manage various medical conditions as well as how patients receive health care. So, what are the distinct advantages to look for when adopting these tech solutions?
Enhanced patient engagement: Understanding how AI is used in patient interactions can help healthcare providers create more engaging and informative experiences for patients. AI-powered virtual assistants and chatbots commonly improve patient engagement by offering on-demand information, answering questions, and facilitating communication with healthcare providers. Besides, personalized AI-driven health education tools can encourage patients to become more involved in taking care of their health with better adherence to treatment plans.
Improved medical care: Progress in generative AI leads to the discovery of novel methods for early disease detection, personalized treatment plans, drug discovery, and more, potentially saving lives and advancing the overall quality of healthcare. This can lead to reduced misdiagnoses, quicker interventions, and ultimately, better patient outcomes.
Cost reduction: Healthcare organizations receive an opportunity to adopt various solutions to optimize their operations. With automated tasks, reduced errors, and improved efficiency, generative AI can help lower service costs and improve resource allocation. For instance, automating image analysis and other data-intensive processes can enable healthcare institutions to see more patients and allocate resources more efficiently.
Streamlined data management: Generative AI is also suitable for streamlining the management of electronic health records. It can automatically extract relevant information from medical records, transcribe handwritten notes, and even suggest treatment plans based on historical data. This reduces administrative burdens on healthcare professionals and minimizes the risk of errors in record-keeping. Its implementation has to adhere to strict privacy and security regulations like HIPAA and GDPR to protect patient data and ensure that decisions remain ethical and medically sound.
Industry progress and innovation: Healthcare is a global concern, and the application of generative AI in healthcare has the potential to benefit people worldwide. Collaboration and knowledge sharing across borders can accelerate progress in addressing global health challenges, such as disease outbreaks and access to healthcare in underserved regions. Learning from successful generative AI examples supports the discovery of best practices and potential pitfalls. This knowledge can be invaluable for researchers, AI developers, and healthcare professionals looking to implement generative AI solutions in their own work.
The profound impact of AI is highly valued across all the domains of the medical industry. It assists involved parties in different tasks and helps to streamline numerous operations and services. Generative AI in healthcare won’t stay behind as it has already supported so many efficient solutions.
HOW TO ADOPT GENERATIVE AI IN HEALTHCARE?
That’s one of the common questions for many businesses that try to identify areas where AI technologies can provide value. Unsurprisingly, the medical industry has become a leader in adopting generative artificial intelligence. More and more teams are working on improving patient outcomes, reducing costs, and enhancing healthcare processes. It refers both to integrating the existing solutions and starting with custom product development from scratch. It is important to collaborate with experts in both AI and healthcare, ensure data privacy and security, and adhere to regulatory guidelines. The ongoing validation and monitoring of AI models are crucial to maintaining accuracy and safety.
So, let’s consider some common use cases for adopting generative AI in healthcare.
Drug discovery
It’s obvious to start with the field of drug discovery as it’s one of the most demanded areas. Businesses tend to invest considerable resources to gain novel insights into the development of medicine. It involves the discovery of new chemical compounds, side effect predictions, optimization of drug properties, drug repurposing, etc. Generative AI accelerates this process and leads to the discovery of more effective treatments while reducing the time and cost of drug development.
Medical image generation
Artificial intelligence techniques are also applied to creating synthetic medical images. It is useful for various purposes, including training models, augmenting datasets, and simulating medical scenarios for research and education. That enhances the quality of medical images, making diagnosing and planning treatments easier for medical practitioners. Teams put considerable effort into training generative models to achieve the required quality while capturing all the specifications of medical images.
Personalized treatment plans
The other generative AI use case in healthcare is related to implementing personalized approaches. It efficiently tailors treatment plans to individual patients by generating treatment recommendations. It embraces all the important details of the patient’s conditions based on their medical history, genetics, and current health status. Seamless integration with existing healthcare systems like hospital management system modules streamlines the decision-making process and supports quality care services.
Healthcare chatbots
Chatbots powered by generative AI can have more natural and context-aware conversations with patients, assisting with appointment scheduling, answering medical queries, and providing health-related information. Healthcare chatbots are available around the clock, offering immediate responses to common medical queries and reducing the burden on medical centers. Besides, this solution can be useful for medical practitioners to receive a more user-friendly and efficient way to access data and handle related operations.
Synthetic data generation
Synthetic clinical data generated by AI can enable researchers to conduct studies and develop healthcare applications while protecting the sensitive information of real patients. That embraces testing and fine-tuning AI algorithms before deploying them in real clinical settings. Synthetic data can be generated quickly, making it suitable for rapid prototyping and experimentation in computer vision, natural language processing, and more.
Healthcare simulation
Healthcare organizations often use generative models to simulate disease progression, patient outcomes, or the impact of different healthcare interventions. That is a valuable base to implement scenario planning and decision support in healthcare management. That allows for a vast range of cases to be simulated, from common medical conditions to rare emergencies. It aids in designing clinical trials, helping researchers determine the optimal parameters and sample sizes for studies.
The market is increasingly fascinated with AI adoption in all healthcare domains. What once seemed beyond the capabilities, now the solutions powered by artificial intelligence find their efficient implementation and integration.
Need professional assistance with AI integration within your organization?
Our team of experts is at your service. Existek specializes in artificial intelligence development services and will gladly assist you with your questions.
CHALLENGES OF GENERATIVE AI IN HEALTHCARE
The sensitivity of this domain underscores the need for rigorous training, stringent regulations, and ongoing efforts to improve healthcare services. Along with implementing generative AI in healthcare, involved parties have to uphold the highest standards of ethics, professionalism, and competence. Let’s consider some urgent considerations and challenges of leveraging generative AI.
Data privacy and security
Healthcare data, including patient records or medical images, must be securely handled to protect patient privacy. Sensitive medical data is always subject to strict privacy regulations like HIPAA, GDPR, and others. Thus, generating, storing, and using patient data with generative AI must adhere to these regulations to ensure data confidentiality. It’s required to implement secure and private model training techniques to train generative AI models without exposing patient data to the model directly.
Ethical concerns
There are ethical concerns regarding leveraging AI in healthcare, including issues related to accountability, liability, and informed consent. It’s essential to mitigate biases and ensure that AI systems provide fair and equitable care recommendations. AI should complement, not replace, human expertise; that’s why healthcare professionals should also be involved in decision-making. By encouraging responsible development practices, like model selection, data collection, and algorithm design, developers are capable of prioritizing safety, ethics, and patient outcomes over speed and profitability.
Validation and regulation
AI models used in healthcare have to go through rigorous validation processes to demonstrate their safety and effectiveness. For example, the U.S. Food and Drug Administration, one of the regulatory bodies, is increasingly developing guidelines for AI in healthcare. The FDA primarily oversees software based on its intended purpose and the potential harm it may pose to patients if it operates inaccurately. As a result, establishing the safety and efficacy of AI systems for medical use requires rigorous testing, validation, and regulatory approval, which can be time-consuming and costly.
Data quality
High-quality, diverse, and representative datasets are necessary for training generative models. It’s worth special consideration to identify and mitigate biases in the data to avoid discriminatory or inaccurate results. Supporting data quality in healthcare is a multifaceted approach, combining data management best practices with ethical considerations and ongoing monitoring and improvement efforts.
Integration with existing solutions
Integrating generative AI into existing healthcare infrastructure can be challenging. Healthcare institutions may need to invest in new technology and adapt their workflows to incorporate AI effectively. For instance, integrating AI into EHRs can be technically complex. Compatibility, data exchange, and workflow integration are essential considerations.
Human-AI collaboration
It’s vital to foster collaboration between AI researchers, healthcare professionals, and regulators to ensure that AI systems are developed and deployed responsibly. This involves training both involved parties and AI models to work effectively together and trust-building between humans and machines. It is delivered through training, trust-building, and defining clear roles and responsibilities. Besides, it is an ongoing process that requires careful planning, continuous improvement, and a commitment to ethical and patient-centric practices.
Despite these challenges, the potential benefits of generative AI in healthcare make it an area of significant interest and ongoing research. Careful consideration and responsible development and deployment of AI technologies are essential to realize these benefits while addressing the associated challenges. Businesses gain definite advantages by leveraging generative AI in healthcare and have efficient means to deal with rising needs.
CONCLUSION
The adoption of generative AI in the medical industry promises to significantly enhance efficiency and transform healthcare delivery. By harnessing the power of artificial intelligence, healthcare professionals can make more accurate diagnoses, discover new treatments faster, and provide personalized care to patients. Additionally, the automation of administrative tasks and improved medical data analysis can streamline operations and reduce costs. However, it is essential to approach the integration of generative AI in healthcare with caution, addressing ethical concerns and regulatory requirements to ensure the safety and privacy of patients. As technology advances, the medical industry’s collaboration with generative AI holds the potential to revolutionize healthcare, ultimately benefiting both providers and patients.
How to develop your AI-powered healthcare solutions?
Contact Existek to discuss your ideas and find an efficient way of software implementation. Our team knows how to turn your ideas into sophisticated products and services.
Frequently asked questions
What is generative AI in healthcare?
Generative AI in healthcare refers to the use of artificial intelligence models, specifically generative models, to create or generate data, content, or solutions within the healthcare domain.
What are some known generative AI examples for the medical industry?
With the great advancements in the AI field, we can observe numerous use cases and impressive solutions to handle various tasks. Here are some of the great examples introduced to the medical market recently.
- Med-PaLM
- AWS HealthScribe
- Oracle Clinical Digital Assistant
- Azure Health Bot
What are the main benefits of generative AI in healthcare?
This advanced tech solution has become really advantageous for different use cases as it embraces better patient engagement, improved medical care, cost reduction, streamlined data management, industry progress, and innovation.
How to adopt generative AI in healthcare?
Adopting generative artificial intelligence in healthcare can offer numerous benefits, including improved diagnosis, treatment planning, drug discovery, and patient care. However, integrating generative AI into healthcare requires careful planning, compliance with regulations, and a focus on patient privacy and data security.